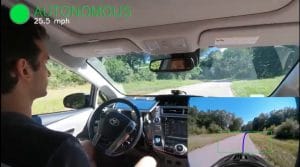
MIT and the Toyota Collaborative Safety Research Center collaborated to produce a photorealistic simulator to help teach AVs to drive in real-world environments.
Researchers at the Massachusetts Institute of Technology working with the Toyota Collaborative Safety Research Center have developed a simulation system with infinite steering possibilities to help autonomous vehicles to learn to navigate real-world scenarios before they venture down actual streets.
The work on the system was done in collaboration with the Toyota Research Institute for its autonomous vehicle research.
While there are plenty of programs designed to help self-driving vehicles to navigate, this new system is called Virtual Image Synthesis and Transformation for Autonomy, or VISTA. It’s a photorealistic simulator that uses realistic images created by artists and others to provide more accurate data.
(Self-driving cars will eliminate only a third of crashes, IIHS claims.)
This data, in turn, is funneled into the simulator, giving the autonomous technology the ability to better deal with sudden, unknown events human drivers face regularly. In recent tests a controller trained in VISTA was deployed in a full-scale driverless car and successfully navigated through previously unseen streets.
The control systems for autonomous vehicles rely on datasets of driving trajectories from human drivers so they learn how to emulate safe driving and to deal with so-called edge cases where events go wrong.
Meanwhile, building simulation engines or virtual roads for training and testing autonomous vehicles has been largely a manual task. Companies and universities use teams of artists and engineers to sketch virtual environments with accurate road markings, lanes, and even leaves on trees.
But the learned control from simulation has never been shown to transfer to reality on a full-scale vehicle, according to MIT’s researchers.
(Americans remain uncertain about autonomous vehicles, poll shows.)
“It’s tough to collect data in these edge cases that humans don’t experience on the road,” said first author Alexander Amini, a PhD student in the Computer Science and Artificial Intelligence Laboratory (CSAIL).
“In our simulation, however, control systems can experience those situations, learn for themselves to recover from them, and remain robust when deployed onto vehicles in the real world,” he said.
But since there are so many different things to consider in complex real-world environments, it’s practically impossible to incorporate everything into the simulator. For that reason, there’s usually a mismatch between what controllers learn in simulation and how they operate in the real world, he added.
Instead, the MIT researchers created what they call a “data-driven” simulation engine that synthesizes, from real data, new trajectories consistent with road appearance, as well as the distance and motion of all objects in the scene.
(AV testing miles in California jumped 40% in 2019.)
The controller also is rewarded for the distance it travels without crashing, so it must learn by itself how to reach a destination safely. In doing so, the vehicle learns to safely navigate any situation it encounters, including regaining control after swerving between lanes or recovering from near crashes.